Lameness detection and scoring
Juan Haladjian 2
Stefan Nüske 3
Dorothée Ledoux 4
David Humphries 5
Lene Munksgaard 6
Isabelle Veissier 7
1 Leibniz Institute for Farm Animal Biology (FBN), Dummerstorf, Germany
2 Technical University Munich, Munich, Germany
3 Ludwig Maximilian University of Munich, Oberschleissheim, Germany
4 Ecole Nationale Vétérinaire d'Alfort, Maisons-Alfort Cedex, France
5 University of Reading, Reading, United Kingdom
6 Aarhus University, Tjele, Denmark
7 Université Clermont Auvergne, INRAE, Saint-Genes-Champanelle, France
Introduction
Enting [1] concluded lameness, from an economic perspective, as the third most costly health disease, following mastitis and reproductive failure issues, in cattle units. Archer [2] estimated the incidence rate of lameness in the United Kingdom cattle herds roughly 50 cases/100 cows in a year; nevertheless, due to poor correlation between incidence rates and records of treatments in farms [3], the actual number seems to be higher. Surprisingly, the significance of lameness associated with cattle welfare, health and profitability of the unit has been greatly underestimated [4]. Recent works have shown a clear link between BCS and hook condition of cows with the development of lameness in these animals [5]. Lameness is a multifactorial and progressive issue where different detriments contribute to its development via complex interactions. Detection of lame cattle can be facilitated through description of the animals' gait characteristics in a numerical scaling system known as locomotion scoring [6]. The total number of visual (manual) locomotion scoring systems can reach up to 25, where differences lie mostly in the used scales, characterization of cows' gait, and posture [7]. Automated locomotion scoring tools would be a big advantage for regular monitoring of lameness in the herd. Three methods that are commonly engaged with automated systems are: kinetic, kinematic and indirect. The kinetic and kinematic approaches measure the forces, involved in locomotion, and time and distance of variables, associated to limb movement, respectively [8]. The indirect method simply exploits behavioral or production data as indicators for impaired locomotion. The automated tools/instruments that will be developed, based upon either of the aforementioned approaches, need to be validated with a ‘reference’ method. This usually is done by comparing with manual scoring; however, it is noteworthy that manual scoring systems have their own set of limitations [9].
Prerequisites
This guideline details steps for detecting and assessing lameness in cattle within the experimental units. These steps are written, presuming that lameness detection is carried out in the units either manually (visual detection) or via automatic lameness monitoring systems. The Animal Trait Ontology for Livestock (ATOL) numbers linked with this guideline are: ATOL_0000835 and ATOL_0001780 (for complete list of ATOL, please visit https://www.atol-ontology.com/en/erter-2/).
A – Lameness detection based on visual evaluation
- The established scoring system within the experimental units should be easy to apply routinely and be time efficient. As recommending a distinct scoring system which is suitable for all experimental units is difficult, use should be limited to just a few systems such as the 3-point scale, e.g. Welfare Quality protocol (http://www.welfarequality.net/en-us/reports) or the 4-point scaling system as recommended by AHDB (http://www.welfarequality.net/media/1121/wqr11.pdf). Regardless of the type of scoring system, it is recommended that the methods used for visual examination are justified according to the literature or in-field practice.
- A systematic lameness assessment of all cows should be carried out every 3–4 weeks (unless the experiment dictates another frequency), whilst any new case of lameness detected in between is also recorded.
- Scoring of dairy cattle should be done after milking, as animals return to the field or housing.
- Operators in the unit should be aware of the close association between lameness and longer lying times and standing in passageway, as well as altered feeding (grazing) behaviour (unless the experiment design assesses other factors). Dairy cows in the unit that display signs of lameness require careful monitoring.
- The surface, animals walk over during lameness scoring, should have a flat and even surface, and should not be slippery or muddy as this will impact gait and lead to inaccurate locomotion scoring.
- Animals should always be evaluated in the same location to reduce variation in scores for repeat observations.
- Ideally, the same operator should locomotion-score the animals from a given herd each time to reduce observer error. If there are several operators, their consistency must be checked regularly (twice a year is recommended). Regular calibration between observers should be carried out.
- The operator should not induce any stress to the animal during the scoring procedure. They should not make any sudden movements or force animals to move forwards. Scoring agitated cows will produce inaccurate scores.
- The operator responsible for locomotion scoring should be trained and retrained by an experienced observer, familiar with the scoring system utilised. This will significantly increase the agreement level between and within observers.
B – Automated lameness detection systems
- Data from automated systems based on visual characteristics of the animal, e.g. change in locomotion or behaviour, must be validated with manual locomotion scoring as a reference (‘gold standard’).
- The automated system should be calibrated according to the reference visual method as stated in point 1 of section A. Users are highly recommended to see the guideline for sensor validation within this chapter of the book.
- Lameness detection with automated tools should be conducted during the normal daily routine (i.e. after leaving the milking parlour) of cows/heifers and ideally without involvement of the operators/staff members (e.g., the platform with loading cells should be placed behind the milking parlor or at the exit to the pasture during grazing season). Animals forced towards an experimental set-up, may hide their weakness or pain due to their stoic nature [10].
- The operator should ensure that dairy cows/heifers do not block the area around the measurement zone when walk-over devices, such as StepMetrix, are being used as this will produce faulty data.
C – Lameness detection using a wearable sensor
The present guideline details steps for collection and data analysis for lameness detection applications. This document has been written, based on a recently developed automated lameness detection system using a wearable motion sensor [11]. The developed system made use of the MicroHub (Interactive wear, Starnberg, Germany). Where the research unit intends to utilise the MicroHub for data collection, a mobile phone is necessary to start and stop data recordings. A detailed description of this lameness detection system has been created by Haladjian [11]. The Animal Trait Ontology for Livestock (ATOL) number linked with this guideline is: ATOL_0000351, AHOL_0005001, EOl_0000001, EOL_0001606, EOL_0001706 and EOL_0001712 (for complete list of ATOL, please visit https://www.atol-ontology.com/en/erter-2/).
- Preparing for data collection: The data collection should be planned in advance, based on the particular purpose of the study, and documented in a collection plan. A data collection plan should include: a) list of materials to be used; b) concise instructions to be followed by the person in charge of the data collection (e.g., how to attach the sensor device, how to orient it, how to start recording data); c) list of actions to be followed during the experiment (e.g., list of cows to be recorded, what needs to be recorded ,and for how long).
- The selection of the cows that will participate in the experiment should be done together with the veterinarian responsible for the cows.
- Modern machine learning algorithms achieve better performances when trained with larger amounts of data. However, cows should not be forced to walk for longer than 10 min per session not only for ethical reasons, but also to avoid a change in the walking pattern due to fatigue. It is recommended that data collection sessions are spread over more than one day for a cow.
- The batteries of devices (e.g., mobile phone, sensor devices) used during the experiment should be fully charged.
- It is recommended that the operator conducts a short (e.g. 10s-long) data collection to ensure proper function of the data collection system (e.g. mobile device and sensor device) and to reduce the chance of human errors before attaching the sensor to a cow.
- Unless the experimental design dictates otherwise, the newly established detection system should be tested on a wide range of animals, based on their physiological conditions (parity, weight, breed, and lactation stage), environmental condition (indoor housing, grazing management), or external parameters (time of the year or day).
- The health condition of the cows selected for data collection should be considered. Cows that are pregnant, undergoing oestrus, or obviously sick (e.g. lame) should be excluded from the experiment.
- Data collection: Regardless of the type of motion sensor used in the unit, the operator should not touch the data collecting device or recording instruments with wet hands. Data collection can be conducted by a single operator if they have experience manipulating cows. However, it is useful to have a second individual to help move cows and restrain them to attach or remove the device.
- The animal must be restrained so that the sensor device can be firmly attached. The optimal place for attachment of the device must be determined, based on the particular application. For lameness, it is recommended to attach the device to the hind left limb as lameness affects mostly hind limbs. How the device is oriented and to which hind limb it is attached does usually not matter, as long as these parameters are consistent across cows and recordings. Figure 1 shows a device attached to a cow and the recommended orientation of the device.
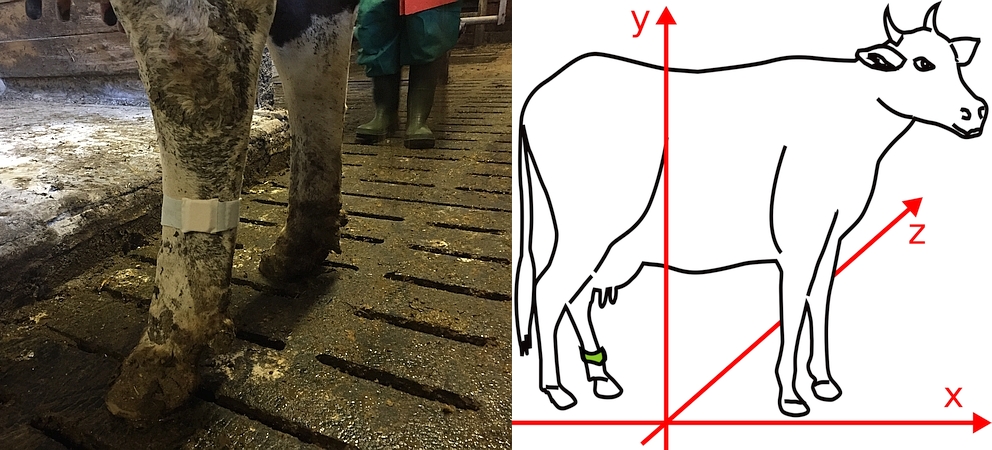
- The animal should be encouraged to walk to allow analysis of its gait. The goal of the data collection is to collect data that resembles the context in which the lameness detection system would be used. When encouraging a cow to walk, the operator should therefore maintain enough distance to the cow, so that it can walk at its own pace. Causing fear or stress to the animal could easily bias the algorithm.
- Researchers should pay attention to the cow’s gaze, particularly when crossing the milking parlour and free pens. Cows will try to enter the milking parlour or free pens to avoid having to participate in a data collection experiment. If possible, the environment should be arranged in such a way that cows walk in a part of the stable that has no access to the milking parlour.
- Operators should document when a cow stops walking for a long time, so that this event can be considered when studying the data afterwards. If a cow enters a pen, it can be encouraged to leave it simply by waving. It is desirable to generate a data set with a similar amount of data across cows. However, only data recorded while a cow walks is useful for lameness detection. Therefore, the data collection should last longer for cows that entered the milking robot or stopped walking for a long period.
- It is often necessary to collect reference data to assess and annotate collected motion data. Some studies may use manual annotation, noting whether a cow walked normally or with a plastic block attached to a hind hoof as a reference. Other studies may require reference values for the entire data set. For example, if a lameness detection system counts the number of strides performed by a cow, reference values for the data would be needed to indicate at which moment in time a cow performed a stride. A common reference data source is video. When using reference data, the motion and reference data sets should be synchronised. This can be done by identifying and matching specific events in the recorded motion and reference data.
- MicroHub: The MicroHub should be turned on by pressing and holding the button on it for 5 s. The light will blink, then display a steady green LED when the MicroHub is turned on. On the app, tapping on the ‘scan’ button will scan for devices nearby with Bluetooth Low Energy (BLE) capability (other BLE devices may show in addition of the MicroHub). From the devices shown, MicroHub should be selected and connected to by tapping ‘connect’. When connected, the MicroHub displays a blue LED.
- Use the ‘start/stop’ button on the app to start recording. When the MicroHub start recording data, it displays a red LED and the app displays the text: ‘Logging Since: sec‘ Once recording, tapping again on the ‘start/stop’ button on the app will cause the MicroHub to stop recording data. Every time, a recording is started, a new file is created in the MicroHub and stored on its SD card.
- Once recording has started, disconnecting the app from the MicroHub will save its battery. When disconnected, the blue LED should turn off. The MicroHub can still record data when it is not connected to the app.
- Before the SD card can be safely removed from the MicroHub, the MicroHub should be turned off. It can be turned off by pressing the white button for up to 4 s. The SD card can be taken out by pushing, then releasing it, and then removing. Data on the miniSD card can be imported to a computer with an adapter. The SD card should contain a text file, named according to the date and time when the recording started. For example, if the recording was started on April 26th 2018 at 14:03 h, the file will be named ‘2018.04.26_14.03.50.969.txt’. The file name should be assessed against the data collection plan and notes taken during the data collection.
- Data analysis: Once data has been collected, an initial check should be carried out to ensure the recording was successful. It is advisable to import the data immediately following data collection, whilst researchers remember the flow of the data collection session (e.g. the order of cows if anything differed from the recording plan). A mistake at this stage could invalidate the data analysis.
- Several things need to be considered when preparing data for analysis: The motion tracker may store data in text format. This is convenient for data collection purposes (e.g. to quickly assess whether data stored in a file is properly stored). However, loading the entire data set in a text format can slow down the data analysis process, as files must be loaded several times. Therefore, data should be stored in a binary format, which will occupy considerably less memory and therefore load faster.
- If Matlab is used for the data analysis, variables in memory can be saved with a simple ‘save<’ command. Before starting data analysis, data should be cleaned up by removing unwanted sections. For example, if the sensor is attached to the cow after starting the recording, data corresponding to the action of attaching it cow should be removed from the analysis.
- Specific gestures (e.g. shaking the sensor), used to synchronise the sensor data to a reference data set, should also be removed.
- Rename data files to uniquely identify a cow and the kind of data it contains in a structured way, so that the different files can be loaded automatically in the script used for analysis. For example ‘cowX_Y’ where X indicates the ID number of the cow and Y the type of recording done for the particular cow (e.g. left to indicate the cow walked with a block attached to its left hoof).
- Researchers should manually test and visualise data processing methods on a small fraction of data firstly. However, to obtain a consistent and statistically significant metric to compare different data processing methods, a metric should be computed automatically for the entire data set. This metric might be the classification accuracy or the F1-score.
- System design: These steps are meant for long-term deployment of a detection system, regardless of the type of sensor utilised. Design decisions should be made, regarding the sensor device, computations that will be executed on the device, and the architecture of the system.
- Energy consumption of the device should be minimised to avoid frequent battery replacements. For this, computation should be done locally on the wearable device and the computed results transmitted, at as sparse time intervals as acceptable for the particular application.
- The sensor should be designed to minimise the risk that the animal injures itself, other cows in the stable (e.g. by knocking the device into them), or the device itself.
- The system should be designed to precisely generate an individual gait profile for each cow and detect changes in their locomotion.
- The system’s data receiver should be installed at the milking parlour. Cattle usually visit the milking parlour at least twice a day and remain still in the same area for several minutes during milking. This period represents an ideal opportunity for the data transmission.
References
[1] Enting H, Kooij D, Dijkhuizen AA, Huirne RBM, Noordhuizen-Stassen EN. Economic losses due to clinical lameness in dairy cattle. Livest Prod Sci. 1997;49(3):259-67. DOI: 10.1016/S0301-6226(97)00051-1[2] Archer S, Bell N, Huxley J. Lameness in UK dairy cows: a review of the current status. In Pract. 2010;32(10):492-504. DOI: 10.1136/inp.c6672
[3] Whay HR, Main DC, Green LE, Webster AJ. Assessment of the welfare of dairy cattle using animal-based measurements: direct observations and investigation of farm records. Vet Rec. 2003;153:197-202. DOI: 10.1136/vr.153.7.197
[4] Van Nuffel A, Zwertvaegher I, Pluym L, Van Weyenberg S, Thorup VM, Pastell M, Sonck B, Saeys W. Lameness detection in dairy cows: part 1. How to distinguish between non-lame and lame cows based on differences in locomotion or behavior. Animals. 2015;5(3):838-60. DOI: 10.3390/ani5030387
[5] Randall LV, Green MJ, Chagunda MG, Mason C, Archer SC, Green LE, Huxley JN. Low body condition predisposes cattle to lameness: an 8-year study of one dairy herd. J Dairy Sci. 2015; 98(6):3766-77. DOI: 10.3168/jds.2014-8863
[6] Sprecher DJ, Hostetler DE, Kaneene JB. A lameness scoring system that uses posture and gait to predict dairy cattle reproductive performance. Theriogenology. 1997;47(6):1179-87. DOI: 10.1016/s0093-691x(97)00098-8
[7] Schlageter-Tello A, Bokkers EAM, Koerkamp PWG, Van Hertem T, Viazzi S, Romanini CEB, Halachmi I, Bahr C, Berckmans D, Lokhorst K. Effect of merging levels of locomotion scores for dairy cows on intra- and interrater reliability and agreement. J Dairy Sci. 2014;97(9):5533-42. DOI: 10.3168/jds.2014-8129
[8] Flower FC, and Weary DM. Gait assessment in dairy cattle. Animal. 2009;3(1):87-95. DOI: 10.1017/S1751731108003194
[9] Chapinal N, de Passille AM, Weary DM, von Keyserlingk MA, Rushen J. Using gait score, walking speed, and lying behavior to detect hoof lesions in dairy cows. J Dairy Sci. 2009;92(9):4365-74. DOI: 10.3168/jds.2009-2115
[10] Weary DM, Huzzey JM, von Keyserlingk MA. Board-invited review: Using behavior to predict and identify ill health in animals. J Anim Sci. 2009;87(2):770-7. DOI: 10.2527/jas.2008-1297
[11] Haladjian J, Haug J, Nüske S, Bruegge B. A Wearable Sensor System for Lameness Detection in Dairy Cattle. Multimodal Technol Interact. 2018;2(2). DOI: 10.3390/mti2020027